일 | 월 | 화 | 수 | 목 | 금 | 토 |
---|---|---|---|---|---|---|
1 | 2 | 3 | 4 | 5 | ||
6 | 7 | 8 | 9 | 10 | 11 | 12 |
13 | 14 | 15 | 16 | 17 | 18 | 19 |
20 | 21 | 22 | 23 | 24 | 25 | 26 |
27 | 28 | 29 | 30 |
- Today
- Total
- activation function
- AdaGrad
- adaptive learning rate
- arithmetic reasoning
- Attention is all you need
- attention mechanism
- auto encoder
- Back Propagation Trough Time
- Backpropagation
- Bayes Theorem
- BCE
- Bert
- Bidirectional Encoder Representation from Transformer
- Binary classification
- BPTT
- Chain-of-Thought
- CNN
- commonsense reasoning
- Computer Vision
- Confusion Matrix
- convolutional neural network
- Cot
- cot reasoning
- counting
- Cross Entropy Loss
- deep learning
- degradation
- Dimension Reduction
- Few-shot
- fine-tuning
목록Cot (2)
데이터 분석 일지
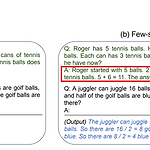
이전 CoT 논문을 통해 pre-trained된 model로 few-shot을 사용하더라도 reasoning ability를 이끌어내는 능력이 중요함을 보였다. 하지만 few-shot을 사용한다면 hand-crafted examplar가 필요하다는 단점이 있었다. 이를 보완하기 위하여 본 논문에서는 answer 전에 "Let's think step by step"이라는 prompting을 추가하여 zero-shot으로도 상당한 성능 향상을 보이며 reasoning ability를 이끌어낸다. 이 기법을 Zero-shot CoT라고 표기한다. 1. Introduction 이전 CoT 논문*에서는 Figure 1과 같이 few-shot을 사용하여 추론할 수 있도록 example을 주었다. 이로써 pre-t..
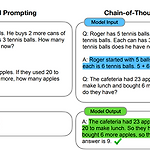
arithmetic, commonsense reasoning, simbolic reasoning과 같이 단순한 모델의 scaling up만으로 성능이 향상되지 않는 task들을 잘 수행하기 위해 본 논문은 Chain-of-Thought를 제시한다. Chain-of-Thought는 natural language task의 결과를 도출하기 위한 추론 단계를 의미한다. 1. Introduction 당시까지는 모델을 scaling up하는 것만으로도 task 수행 능력이 향상하였다. 하지만 단순 예측에서 끝나는 것이 아니라 단어의 의미를 이해하고 추론해야 하는 task들은 단순 scaling up에도 충분한 성능 향상이 일어나지 않았다. 본 논문은 이런 task들로 artihmetic, commonsense ..