일 | 월 | 화 | 수 | 목 | 금 | 토 |
---|---|---|---|---|---|---|
1 | 2 | 3 | 4 | 5 | ||
6 | 7 | 8 | 9 | 10 | 11 | 12 |
13 | 14 | 15 | 16 | 17 | 18 | 19 |
20 | 21 | 22 | 23 | 24 | 25 | 26 |
27 | 28 | 29 | 30 |
Notice
Recent Posts
- Today
- Total
Tags
- activation function
- AdaGrad
- adaptive learning rate
- arithmetic reasoning
- Attention is all you need
- attention mechanism
- auto encoder
- Back Propagation Trough Time
- Backpropagation
- Bayes Theorem
- BCE
- Bert
- Bidirectional Encoder Representation from Transformer
- Binary classification
- BPTT
- Chain-of-Thought
- CNN
- commonsense reasoning
- Computer Vision
- Confusion Matrix
- convolutional neural network
- Cot
- cot reasoning
- counting
- Cross Entropy Loss
- deep learning
- degradation
- Dimension Reduction
- Few-shot
- fine-tuning
목록Paper Review/Computer Vision (1)
데이터 분석 일지
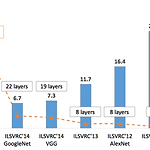
일반적으로 네트워크가 깊을수록 학습이 어려워지고, gradient vanishing 문제가 발생한다. 본 논문에서는 충분히 깊은 DNN에서도 학습을 쉽게 하기 위하여 residual learning이라는 개념을 도입한다. 각 layer마다의 입력과 출력의 차이인 residual을 학습하여 더 쉽게 최적화할 수 있는 방법이다. 이 개념을 도입하여 본 논문에서는 152개의 layer를 쌓아도 낮은 복잡도를 가지게 된다. 1. Introduction Deep convolutional neural network가 이미지 분류 task에서 점점 발전하고 있다. 또한 network의 depth는 학습하는데 있어서 굉장히 중요하다. layer를 많이 쌓을 수록 성능이 좋아진다는 것을 의미한다. Figure 1을 보면..
Paper Review/Computer Vision
2024. 3. 25. 19:18