일 | 월 | 화 | 수 | 목 | 금 | 토 |
---|---|---|---|---|---|---|
1 | 2 | 3 | 4 | 5 | ||
6 | 7 | 8 | 9 | 10 | 11 | 12 |
13 | 14 | 15 | 16 | 17 | 18 | 19 |
20 | 21 | 22 | 23 | 24 | 25 | 26 |
27 | 28 | 29 | 30 |
Notice
Recent Posts
- Today
- Total
Tags
- activation function
- AdaGrad
- adaptive learning rate
- arithmetic reasoning
- Attention is all you need
- attention mechanism
- auto encoder
- Back Propagation Trough Time
- Backpropagation
- Bayes Theorem
- BCE
- Bert
- Bidirectional Encoder Representation from Transformer
- Binary classification
- BPTT
- Chain-of-Thought
- CNN
- commonsense reasoning
- Computer Vision
- Confusion Matrix
- convolutional neural network
- Cot
- cot reasoning
- counting
- Cross Entropy Loss
- deep learning
- degradation
- Dimension Reduction
- Few-shot
- fine-tuning
목록BPTT (1)
데이터 분석 일지
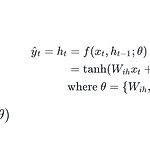
0. Foundation Concept of RNN 이전까지는 함수에 x를 입력 받으면 y를 출력하는 형식을 정의했었다. 하지만 RNN은 sequential data나 time series data를 다룰 수 있는 구조를 지닌다. RNN은 이전 time step의 출력을 입력으로 받는 특성을 가진다. 따라서 x와 이전 time step의 출력 h_(t - 1)을 입력으로 받아 현재 time step인 h_t를 출력하는 구조인 것이다. FC layer나 CNN은 이전의 출력 정보를 입력으로 받지 않고, 순서 정보를 고려하지 않는 반면에, RNN은 순서 정보나 이전 데이터에 기반한 현재 데이터의 처리를 할 수 있다. 0. 1. Sequential Data vs Time Series Data sequen..
Lecture or Textbook Review/Deep Learning
2024. 3. 7. 11:54